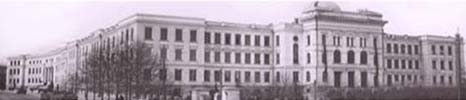
Cluster Analysis for the Assessment of the Avoidance Behavior
Author: Nino ArchvadzeCo-authors: Sulkhan Tsagareli, Otar Tavdishvili
Keywords: Cluster Analysis, "Behavior vector", Avoidance Behaviour
Annotation:
Nowadays a wide range of mathematical methods gain a prominent position in biological sciences. Mathematical methods are applied for neural network modeling, assessment of: behavioral strategy in adaptive learning and decision-making tasks, reinforcement and strategic changes; for ecological modeling etc. Through our study we examine and confirm possibility of application of unsupervised cluster analysis for multi-parameter assessment of behavioral conformities through avoidance acquisition in different experimental groups of albino rats. The measured behavioral parameters are: 1) reactions to the light—avoidance reactions; 2) reactions to the painful foot-shock - escape reactions; and 3) inter-trial spontaneous behavior (jumping onto shelves). Three different groups of 31 albino rats of both sexes (average body weight of 150-200 g) were examined. The animals were divided into three groups designated as Group A (intact; n = 13), Group B (Neocortex Coagulated -NCC; n = 9), and Group C (Dorsal Hippocampus Coagulated –DHC; n = 9). For partitioning of rats according to their behavioral similarities the unsupervised clustering algorithm based on Parzen statistical estimation of probability density function were applied. Method considers the case, when both the probability density of an initial data set and the number of data classes preliminary are unknown. It gave us possibility to classify rats’ behavior by their active avoidance acquisition ability. The term “behavior vector” for quantitative description of behavior in learning process was introduced. The behavioral parameters (features) getting different numerical values during the experiment compose the components for the “behavior vector”. Such approach enables to classify the animals by their learning abilities into classes (groups) according to the degree of behavioral similarity. So, as a result of classification we’ll obtain classes in which elements of the "behavior vectors set will arrange into groups in order of magnitude of the Euclidean distances between them respectively. Consequently, several classes (groups) of animals with different behavioral capabilities were identified and each group included the animals with similar behavio¬ral features. This first class, value of which identifying cluster’s number l, involved rats with most resembling behavioral patterns and every next class exhibited less similarity to it. Distribution of the extracted classes of rats from different test groups was defined. The class number change dynamics and relative frequencies of homogeneous classes were assessed. Obtained results logically matched with progress in learning process. The high relative frequency of appearance of class 1 significantly differed from the other. The groups with prevalence of animals of class 1 were revealed and assessed in percentage. The individual learning ability of each subject involved in class 1 was assessed. Proceeding from the analysis it was established that 24% of intact, 35% of NCC and 26% of DHPC rats were not included in the first class. Only 9.67% of intact animals best succeeded at avoidance behavior. No animals among DHPC or NCC groups could achieve such levels. 3.22% of intact, 9.67% of NCC and 6.45% DHPC rats were found to be good at learning. Lower learning ability was revealed among 25.8% of intact, 12.9% of NCC and 6.45% with DHPC. We have demonstrated compliance of our approach simplifying capability to reveal similarities in biological studies throughout multi-factorial assessment. The proposed method is convenient to assess individual variation also. The proposed approach represents our attempt and wiliness to profound multidisciplinary approaches in further research.
Lecture files:
კლასტერული ანალიზის გამოყენება თავდაცვითი ქცევის შეფასებისათვის [ka]Cluster Analysis for the Assessment of the Avoidance Behavior [en]